S5 Ep 3. Steve Escaravage: Executive Vice President at Booz Allen
- 0.5
- 1
- 1.25
- 1.5
- 1.75
- 2
Tim Schigel: Welcome to Fast Frontiers. I am your host, Tim Schigel, managing partner of Refinery Ventures. In this episode, we're talking with Steve Escaravage, the executive vice president at Booz Allen, where he leads a team of 4, 000 professionals in their analytics practice and artificial intelligence services business. They assist their clients with operational integration of data science, machine learning and AI solutions. In today's episode, we're going to talk about pop cultures, ideas of AI, and whether people should be afraid of this technology. We'll look at comparisons of state- of- the- art AI applications between government sectors and commercial businesses and how Booz Allen thinks about venture back startups and opportunities to collaborate with them. The biggest theme or, so what, I hope you can take away from this conversation is Steve's advice for sourcing and innovation and talent, which is to read the national Security Commission report on artificial intelligence. Please enjoy my conversation with Steve Escaravage. Steve, welcome to Fast Frontiers. It's great to have you with us.
Steve Escaravage: Thanks for having me, Tim.
Tim Schigel: You have incredibly fascinating job and role at Booz Allen. A couple big things we want to cover today, but first of all, can you just share a little bit about your, you've been there a long time, Booz Allen, for those who don't know about Booz Allen and what your group does specifically?
Steve Escaravage: Yeah, absolutely. So thanks again for having me. So let's start with Booz Allen and I can go into a little bit of my role there. So Booz Allen is a hundred year old plus company that really started management consulting as a field, as a profession. Started in Chicago long time ago. And what we basically do is we provide consulting services largely now to the federal government, but we have a rich history of supporting top Fortune 500 companies around the world. And we still have a thriving commercial business, although it's smaller than our pretty substantial federal business. And so all of the executive departments of the governments and the agencies that you might be familiar with, just like other large corporations, they need help accomplishing their technology and strategic objectives. And so Booz Allen is a professional services and technology provider to those agencies, about 29,000 people, almost a$ 10 billion business on last check. And we really support all of the missions of the federal government in some shape or form just given the size of the company. And so we support the executive departments on the civilian side, health and human services, Department of the Treasury, the defense side, Department of the Defense. We support all of the armed services. We've been doing so since the 1940s. And then on the national security side, we support many of the intelligence agencies who provide that essential mission. Think of Booz as providing analytics, engineering, digital, cyber. Those are the core constructs of the services that we provide. And my specific role is, I lead Booz Allen's analytics and AI business. So we have over 4, 000 analytics practitioners at Booz Allen, applied mathematicians, statisticians, computer scientists, and within that, we have over 650 practitioners of artificial intelligence. These are machine learning engineers, these are advanced data scientists. We're building AI solutions, implementing AI systems to help solve some of those problems. And that aligns with my background. My background's in applied mathematics and computer science. Tim, as you mentioned, I've been in this game now for over 20 years and I'm excited that maybe finally, in some ways we have all of the ingredients coming together so that we can really provide revolutionary gains in these fields and improve upon evolutionary capabilities that came before.
Tim Schigel: How long has this AI group been together? When did it really start as AI, not just analytics?
Steve Escaravage: Oh, that's a great question. A lot of people think about this current AI wave and they experienced the big data analytics wave that happened in the middle of the 2000s, 2005, 6, 7, 8. We started hearing a lot about big data analytics. And then a little bit later in the decade, we started hearing about data science and it was named the sexiest job in America and got all this inaudible. And now AI, here we go, artificial intelligence. In reality, in the worlds that we support, especially in defense and national security and healthcare, AI has been underway for quite some time. We have programs that we support where we've been using machine- learning based solutions and AI, non- machine learning based AI solutions, for decades. But the team at Booz Allen really was founded in the 90s by a few brilliant people who, gentleman by the name of Mark Herman, who was really a visionary in the area of war gaming. And then gentleman by the name of Bill Fillet, who was a brilliant mathematician. And these two folks came together, right at around that time in Washington, DC, where we were trying to figure out, how do we think differently about the future of national defense and security and how do we use analytics to help inform the decision making around that. And they came together and really wrote some incredible pieces that served as the foundation for some new thinking around these areas. And so to answer your question more briefly, this business was really founded in the 90s in the areas of optimization and modeling simulation using machine learning and analytical methods. And then really when this AI wave picked up, it's probably about five to seven years ago is where we had started investing heavily in AI, and in some ways that timing helped us achieve an early mover advantage on our field.
Tim Schigel: So when you and Booz Allen think of AI, start with the basics, how do you describe it?
Steve Escaravage: This is the great debate, right? We describe AI as an outcome and it's when we use machines to perform tasks that we would normally require human level intelligence to complete. And so if you think of it as achieving an outcome, you've achieved artificial intelligence is the way that I like to think about it, but at the same time, it's also a field of research. And in many cases, people will say that thing, that system, is AI, and that's okay in my book as well too, you can have an AI system which implements methods and techniques that allow you to achieve that outcome of artificial intelligence, able to perform tasks that normally would require human- level intelligence. Does that make sense?
Tim Schigel: Yeah. Very good. Very good. And so sticking with popular culture, ideas of AI, should people be afraid of AI?
Steve Escaravage: I think that there's more upside. This is the thing that is really frustrating that we see in the news media today is, unfortunately, I don't know if it's human nature or what it is, but we're all drawn to the negative, when in fact, the upside related to this technology, and most emerging technologies, is hard to fathom. The downside, I think, and the risks, there are risks associated with large scale enterprise adoption at AI that have been well documented and well discussed. What I wish though, was the upside, the benefit to individuals from personalizing their experience in my world, the ability to change from an opt- in to an opt- out model around some government services and entitlement benefits, because we can infer from the data that you qualify, the ability to personalize everything we do in day to day life, the ability to make transportation safer, energy more efficient, actually security and the national defense safer through AI that I wish we talked more about that. And I believe the upside far, far outweighs the risks. There's still risks though that we need to manage.
Tim Schigel: What would be those risks?
Steve Escaravage: For the first time in probably, maybe human history, we have a technology that is going to scale at a pace that's hard to imagine. It has already happened in many of the commercial arenas that we deal with and we don't even know it. If you think about how quickly GPS navigation and the fact that we don't use maps and that we use apps and we trust them, it wasn't that long ago that we didn't do it that way. But I think the risk is that with this incredibly powerful technology, it's the scale that is possible, and it's the pace at which it's going to move out, that we have to be careful to engineer the quality and responsibility into the process by design and not try to retrofit it on at the end. Because like we did with cyber in internet technologies where it was a bit of an afterthought and we had to retrofit it on at the end, it will be hard to do that because these machine learning- based AI solutions in particular are not as intuitive. And so there is potential risk for inequities, there is potential risk for unintended outcomes, and it would be better if we can engineer to manage that risk upfront, instead of trying to chase it on the backend.
Tim Schigel: How do the commercial and federal or government sectors compare in terms of state- of- the- art application of AI?
Steve Escaravage: Yeah, this is always pretty shocking to me. I've worked on both sides. I spent the beginning of my career working in military intelligence and defense and then spent a good bit of the middle of the road working in commercial, with pharmaceutical companies, oil and gas companies, financial institutions, large scale transportation infrastructure companies on the commercial side. And there's always this belief that the other side is doing things that we're not doing. I've been in some of the largest companies and they're really interested in understanding what is the major go programs? Behind all the super secret classified worlds, what do you see? And there's some pretty incredible technology being implemented in that space. And then I've been in federal agencies who are executing a mission that has an adjacent mission in the commercial space, and I've seen what we're able to do on the federal side and I go into these Fortune 500 companies and I'm blown away by the degree of technology innovation that they've had. And so I think that in some ways it's comparable in that there's pockets of just amazing technology and even more amazing adoption of that technology. Where they tend to differ though, is on the government side, given the sensitivity of the mission, the security architecture, the discipline of getting it right by design, the risks are so high that in some cases you can't iterate your way to trust because they're for in inaudible benefits serving 30 million people, they are payment programs in the billions of dollars of programs, they are dealing with national security information that we certainly need to protect. So on the government side, the process, the security requirements, the necessary steps to implement technology, there's a very high bar. And in some cases there's a lot of criticism around that.
Tim Schigel: If we fast forward in 20 or 30 years, is it likely that we'll look back at this time and say, Hey, this is the time when either great advances in AI, cybersecurity, et cetera happened because of that focus and need?
Steve Escaravage: Yeah. We talk a lot about this and I do believe that there's been an inflection point and that most of the breakthrough innovations, those examples that we've talked about historically have come from initial government funding, which then drove commercial industry adoption. I do believe that that inflection point has happened in that the investment in commercial industry, in private industry, is where the breakthrough innovations are occurring today. I do hope that through sustained federal or government funding there can be a balance there because there will always be some missions that the federal government hopefully can incentivize the innovation corridors to care deeply about. And I think the National Security Commission on Artificial Intelligence gave an incredible perspective on this, is that we simply can't, from a federal standpoint, ignore just the magnitude of investment in commercial industry. That's where the breakthrough innovations will come in the future. At the same time, there's an opportunity for the federal government to help concentrate that energy and provide channels to help close that gap. But it shifted over the last 30 years, and that's going to be interesting to watch in the future.
Tim Schigel: So back to thinking about AI and where we are in its life cycle, and you think about general AI or artificial general intelligence we're still nowhere near computers and machines having consciousness. I think sometimes people maybe who don't understand the technology and how it works, missed that point. So where would you say, or which inning are we in the development of AI?
Steve Escaravage: Yeah, I like to think about it on the, one of the hype cycles is, I ask myself in this current wave of AI, are we at that peak hype or are we a little bit past it? I actually feel like we maybe a little bit past it, and we're coming up on that trough of disillusionment that will happen where a lot of investment has happened and hopefully though, based on learning from previous innovation waves, that trough won't be so deep related to AI. Because the evidence is out there, in my belief in terms of, it is time to press the, I believe button. We've seen enough in the academic literature, we've seen enough in implementations where people are getting real value. But I do think we're somewhere in that window, right around peak, maybe a little bit past it. And there will be time in the years ahead where people will start asking questions of the amount of investment that's been made and the return that we're seeing. Again, I think that the potential is very, very high. And then back to artificial general intelligence, not only is that something that we still don't know if we will achieve, there is not a pathway there today based on the methods and techniques that have been published and reading some recent books, I really think that again, there's more upside and the potential for humanity for all of the institutions we care so much about over the next 20 to 30 years is where I think we should have the focus. And then we'll see if we have a number of revolutionary inventions that even give us a pathway towards AGI and my opinion.
Tim Schigel: Well, one of the things you mentioned a couple minutes ago, that's key to this is scale, right? The ability to learn et cetera, it leverages and needs to take advantage of scale. So very important component, both scale in terms of distribution, as well as the scale of data, right? How do you, your group and Booz Allen, think about when you're looking at technology and trying to understand the industry, how does a large from like Booz Allen think about venture back startups and what should entrepreneurs be thinking about as they think about the work that you're doing and whether or not there's opportunities to collaborate?
Steve Escaravage: The thing that probably changed most over the past decade in my world is we largely, because of the sensitivity and the classifications of some of the programs that I work on, you had to almost have chain of custody around every line of code that was written in solutions. And what resulted was this break between what we all saw on our day to day lives and the commercial worlds we lived in and what we were reasonably able to build in real time on these highly sensitive programs. And about a decade ago, we realized that this wasn't going to scale over time, that the plots were moving at different slopes, and that we needed to find a way to bring in commercial technology into the sensitive missions of the government. Fast forward 10 years and I have never been more convinced that the rate of change of all of these emerging technologies makes the whole ecosystem around the federal enterprise really dependent on breakthrough innovations coming from the innovation corridors into this space. And I like to talk about, we used to typically think, build first, as a mentality, and now it is, apply first, as a mentality. And that shift happened within the last five years when secretary Ash Carter in 2016 really started a trend in the defense and Intel space. And I think it's really moved across federal government now. But when, when I think of venture backed startups, I always as an integrator and as somebody who has folks with deep expertise in the mission and deep expertise in these emerging technologies and how to engineer those to the last mile per problem of the customers that I solve. We're always looking for folks who specialize in different areas of our technology reference architecture who can come in and basically close technical debt to help solve a mission. And we've had incredible success in partnering with companies that were very early in their maturity and now they're massive companies. I think about our relationship with Databricks, as a company where I remember meeting them a number of years ago and was really intrigued by the business model that they were bringing forward, and the fact that they had this highly adopted open source software that they were going to then provide enterprise support to. And I thought that was an intriguing business model and it's been incredible to watch them. And there's so many examples where we have partnered with companies in that space and they've brought incredible value to the missions that we support. And there is a dependency there as a result. As an integrator, I want to bring the best technology to my clients. And in almost every case now we're looking into the innovation quarters as a first step.
Tim Schigel: Do you find that the startups are... How far along are they and do they need to have dedicated, federal business development resources on their team, or do you help them bridge that gap?
Steve Escaravage: A little bit of both. In some cases I think the companies who have really excelled is companies who do have folks with experience in our sector. Just like anything else, if you were going to push into a new region of the world or a new industry, if you're a horizontally- focused business or utility- based business, you bring somebody in with expertise in that area. We have found that when we can communicate with somebody on the side of these startups who has experience working with federal contracts, who mostly understands the regulations as a regulated industry that we deliver in, that is very beneficial. But in some cases we help bridge that gap where again, we've signed on to help integrate a system of systems to generate some outcome and we will help companies figure out how to navigate that space. We've been doing it for over a hundred years. We've got a lot of experience helping companies bridge that gap.
Tim Schigel: So what are some areas that you are actively looking for solutions or opportunities that you want to get in contact with some of those entrepreneurs?
Steve Escaravage: In AI right now there's a few, probably major technological hurdles that we're seeing now, as we enter this state of the technology. At first, it was all about standing up pilots that could convince people of the potential of AI and so you saw a lot of computer vision pilots, a lot of natural language processing pilots, and they were pilots. And to a certain extent, I like to say we got stuck in pilot purgatory, where everybody had pilots and then we're looking for the cumulative impact to these organizations and these missions. And it's hard to get examples where you get scaled implementations. And we found three things were always the longest pole in the tent. One was the access to data problem is, how do you get access to data? How do you do so in a secure manner? How do you do so in compliance with data license agreements? There is restrictions around aggregating certain types of data sets in the areas that I work from a classification standpoint, but also from law statutory and regulatory and policy. And so technologies that help solve the data problem. There's a lot of excitement around using synthetic data as a source to train AI models. And I'm blown away by the literature, it actually works. Also been really impressed by some of the companies and partners that we work with that have scaled data labeling enterprises and annotation.
Tim Schigel: Yeah. I'm surprised by that as well. Synthetic data that at first you're like, how's that going to make sense?
Steve Escaravage: It makes sense. If you really take a step back and think about how techniques like machine learning works and how it tries to reduce the dimensionality of the problem, and then take all of these inputs and tune them to improve a prediction, improve an outcome. If we think about synthetic data operating in a lower fidelity reduced dimensionality space, there's ways to start to wrap your head around, Okay, well, that makes sense why that would work. But I'm still blown away by, you can see where training models now on what looks like bad inputs from video games in the late 80s and early 90s. Sure enough, when you try in real world settings, it works. And I think that the irony in that is great, because it's a little bit of nostalgia for us, but it works, which is really exciting. So I think that's one area. The two other areas that we see is, to get adoption you have to have trust, you have to understand how these systems are being engineered, you have to understand the reproducibility of the systems and the sustainability or maintainability of these systems. And so we've published some material on the importance of AI or machine- learning operations, and that end to end process of applying best practices for system engineering and software engineering, but then being able to monitor the behavior of these AI systems in production and understand where additional calibration and tuning is needed and how to complete that feedback loop from the R and D environments into production to constantly improve the systems that are deployed and doing so in a manner with transparency and an approach to get at that ethical adoption or responsible adoption of AI to really understand what unintended outcomes could be for present, what bias might exist in data or systems or implementations and how do we measure it? That whole piece around responsible AI in the operations is a second area of a lot of interest.
Tim Schigel: That reminds me of the black box problem that people talk about, Hey, you may be coming up with the right answer, but I don't know why. I heard it articulated in healthcare, where doctors want something that's clinically intuitive. If they're going to get assisted by something, by some intelligence, it needs to be clinically intuitive, so it needs to relate to their understanding of biology and human systems. So in your mind, is that related? Are they the same thing to the trust issue or is it something different?
Steve Escaravage: I think there's a couple different angles at it. There are some methods in the family to achieve artificial intelligence outcomes that are more explainable than others. Classic example is, if we build a decision tree that we implement, that is some rules that's used to make a decision, you can go back and walk down the decision tree and understand why one outcome was predicted over another. It is difficult to do that in some of the advanced neural network based solutions where you can have some level of insight, but intuition is hard to understand exactly why in all of these coefficients and all of these layers that we're getting outputs that we're getting. So I think part of it is actually being able to trace inputs to outputs and there's a trade off to be had there. I think the other piece is that because these systems are complex and you have data of unknown provenance and unknown lineage, in many cases you have systems that are just beyond human intuition, to take a step when they're operating, you have to inspect them to understand exactly what is taking place. And they're deployed in environments that are constantly changing. It is as dynamic an environment as you can. And the fact of the matter is that there is always bias in data, how it was sampled, individual methods and techniques have their own analytical bias, and there is real risk when mathematical bias aligns with social bias that we have to find a way to manage and eliminate from systems. Otherwise, people won't trust them and we won't get that upside that we talked about.
Tim Schigel: So what's the third? So first one was access to data. Second was trust. What's the third?
Steve Escaravage: Most of the AI problems that people study today, they study in pristine computing laboratory environments. They write awesome papers that we all get super excited about. The reality is that most AI systems are implemented at the edge. And so this era of IOT, this era of at- the- edge is where many of these systems need to be implemented just based on the underlying infrastructure, the latency that needs to be achieved and user preference. People like their phones. They want to be able to run these systems, these algorithms, these models on the devices that they want in a disconnected fashion. And that's what the user prefers. And so we have to find ways to solve it. In the area that I support in defense national security, healthcare, even in some consumer applications, if you cannot replicate the success of the research environment at the edge, you've not solved the problem.
Tim Schigel: So as you think about the sourcing of innovation and talent, what is important about the time that we're in now?
Steve Escaravage: Yeah, great question. I would encourage all the listeners to go take a look at the National Security Commission on Artificial Intelligence, released the final report after study. And I believe that this is the most important document that will be written around in this space with regards to how AI can benefit the federal mission and what we need to be doing for at least the next decade, if not longer. It's an incredible piece of work. But in there it really lays out a call to action to industry in our country around understanding and supporting the public sector mission that of the government. There's been well documented, well publicized cases over the last decade where the innovation quarters and Silicon Valley, maybe were diverging a little bit from core support of the federal mission and I just tell you, we need help. This is an interesting time in the history of our country and in missions that we're supporting, given all the world inaudible events. We need the innovations coming from the commercial sector and private industry into the federal mission. I think the government has done an excellent job of creating new pathways to make that happen, but as a leader in the industry who's in this space, I spend a lot of my time trying to help companies understand the potential for their businesses and for their investors and their stakeholders in the public sector mission and I'm always open to having conversations around that because I really do believe that if you're a fan of democracy and you care about this country, we're going to need to support our federal government in the adoption of AI and other emerging technologies. We need the help of private industry to do that. So I look forward to engage aging with companies in your fund and also more broadly.
Tim Schigel: Awesome. It's a great time to be an entrepreneur and a patriot. Thanks for that call to action there, Steve, and thanks for being on Fast Frontiers.
Steve Escaravage: Thanks again.
Tim Schigel: Join us next week when we bring you my conversation with Carl Grant, executive vice president of global business development at the Cooley Law Firm. Thanks for listening to Fast Frontiers. If you like our show and want to know more, check out our website, fastfrontiers. com. If you enjoyed this episode, please share it with others and leave us a review on your favorite podcast platform. The Fast Frontier's podcast is brought to you by Refinery Ventures. Our producer is Abby Fittes. Audio engineering by Astronomic Audio and our podcast platform is Casted.
DESCRIPTION
Steve Escaravage, Executive Vice President at Booz Allen, talks about what A.I. is and its uses at Booz Allen, as well as popular ideas surrounding artificial intelligence. Tim and Steve also discuss how entrepreneurs can approach opportunities to collaborate with venture capital firms, hurdles to A.I. development, and Steve's thoughts on where the technology is headed.
Today's Host
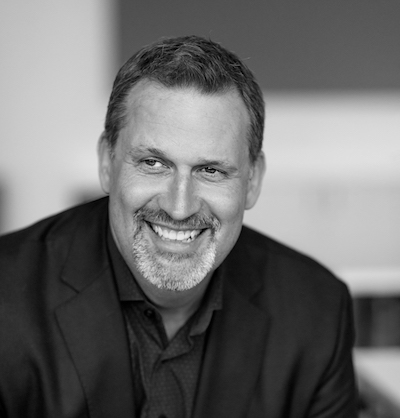
Tim Schigel
Today's Guests
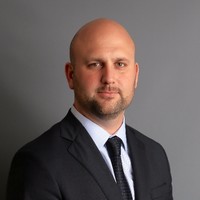